Pozvaní prednášajúci
Lubomír Soukup (ÚTIA): Jak jsou přesné digitální mapy?
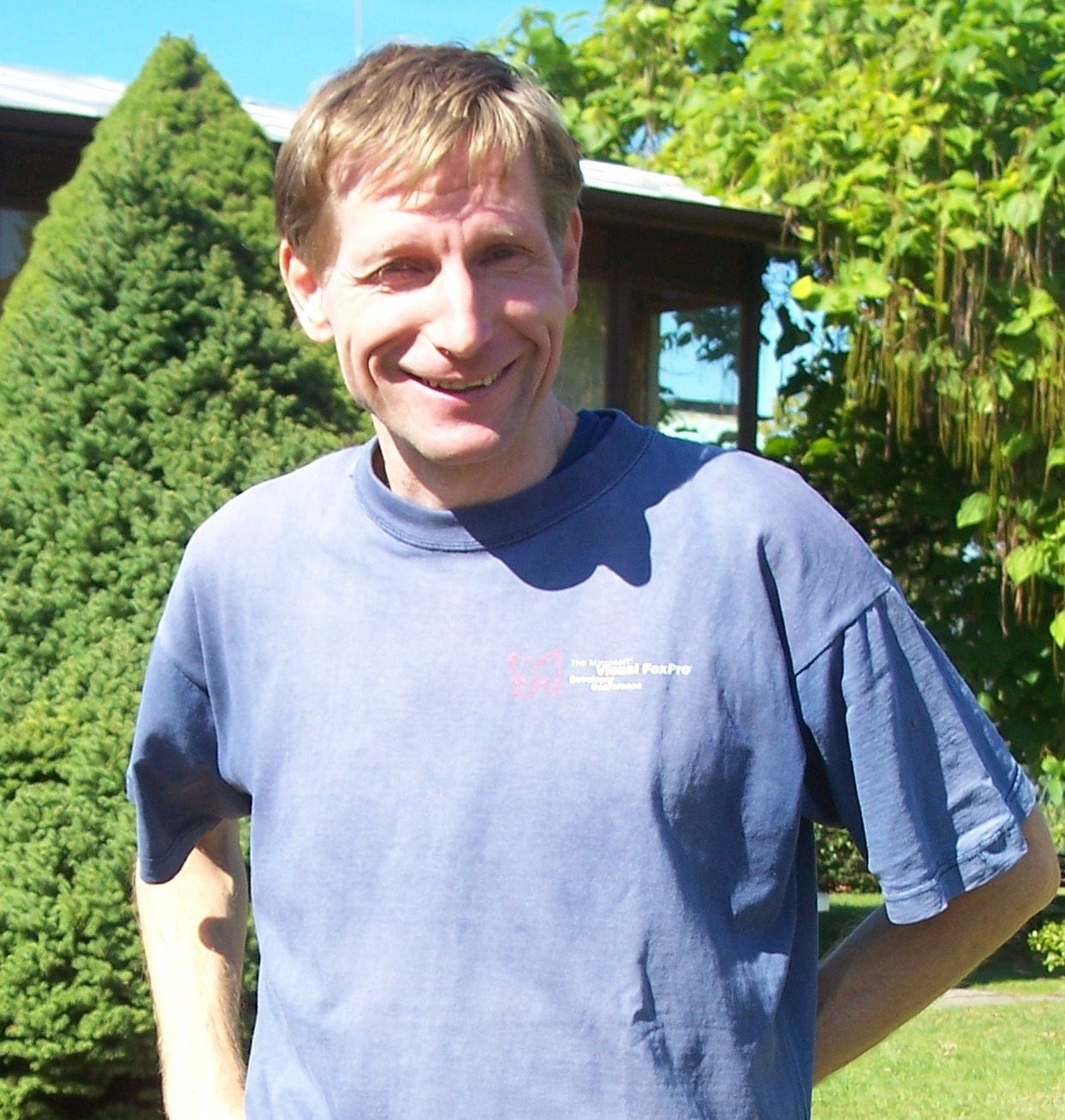
Na přednášce bude představena metodika tvorby digitálních map sestavených z různých datových zdrojů. Pozornost bude věnována polohové přesnosti digitálních map, která je významná zejména při porovnávání obsahu starých map se současnými mapami. Poukážeme přitom na souvislost se zpracováním digitálních obrazů i na specifika zpracování starých map a glóbů.
Lubomír Soukup absolvoval inženýrské studium na Stavební fakultě ČVUT. Jeho studijním oborem byla geodezie a kartografie, specializace dálkový průzkum Země. Pracoval ve Výzkumném ústavu geodetickém, topografickém a kartografickém, potom na Stavební fakultě ČVUT v Praze a nakonec v Ústavu teorie informace a automatizace AV ČR, kde působí dodnes. Výzkumný zájem Lubomíra Soukupa se soustřeďuje na matematicko-statistické metody zpracování prostorových dat, zejména na geostatistické metody a bayesovský přístup. Tyto metody aplikuje na digitální obrazy a mapy. V současné době se zabývá především elastickou registrací (vlícováním) digitálních obrazů. V posledních letech se podílel na projektu "Výzkum možností pozemního InSAR pro určování deformací rizikových objektů a lokalit", v jehož rámci vyvíjel algoritmy na zpracování dat z interferometrického radaru (InSAR). Výsledky tohoto projektu byly oceněny Cenou inovace roku 2016.
Jiří Hana (Geneea): Generování textů v přirozeném jazyce
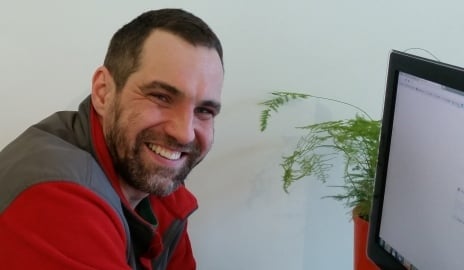
Přednáška představí problematiku generování textu na příkladu sportovních zpráv. Jde o společný projekt nakladatelství Economia a firmy Geneea. Vyvíjený systém vytváří novinové články popisující jednotlivá sportovní utkání. Články jsou generovány na základě strukturovaných dat o událostech na hřišti a dat v databázích, jakou je například znalostní báze Wikidata. V této fázi projektu jde pouze o fotbalové zápasy, ale v blízké budoucnosti bude systém rozšířen na další sporty a výhledově také na zprávy o počasí a zprávy z burzy.
Jiří Hana má zkušenosti jak z firemního, tak z univerzitního prostředí. Vystudoval informatiku na Matematicko-fyzikální fakultě UK a lingvistiku na Ohio State University. V USA strávil téměř 10 let. Mezi jeho zájmy patří morfologie, příklonky a jazyk cizinců. Je výzkumným pracovníkem na Ústavu formální a aplikované lingvistiky MFF UK a spoluzakladatelem společnosti Geneea Analytics, startupu vyvíjejícího systémy pro analýzu a generování textů.
Antoni Ligęza (AGH University of Science and Technology in Kraków, Poland): Rules, Causality and Constraints. Model-Based Reasoning and Structural Knowledge Discovery
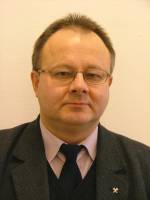
Data Mining techniques are widely applied to build models in the form of rules, decision tress or graphs. Some most successful techniques include algorithms for decision tree induction (with ID3, C4.5, C5.0 being the most prominent examples), frequent pattern mining (e.g. the Apriori algorithm for association rules mining) or Directed Acyclic Graphs for causal probabilistic modeling (the Bayesian Networks). In the domain of Fuzzy Sets there are approaches covering the experimental data (e.g. the Hao-Wang algorithm). Some more mathematically advanced tools incorporate Rough Sets Theory, Granular Sets, or approximation tools.
Such techniques, although useful in practice, are limited to discover the shallow knowledge only. They are based on efficient grouping techniques, relative frequency, or estimated probabilistic distributions. In general, they quite often answer the question “how does the system behave?” in terms of input-output relation, but unfortunately do not explain “why the systems behaves in a specific way” --- with reference to it internal structure and components.
In contrast to widely explored popular Data Mining tools and techniques, the presentation is focused on investigating the phenomenon of causality and exploration of the paradigm of Model-Based Reasoning. An attempt is made to describe the idea of causal rules and functional dependencies on strictly logical background. The main focus is on modeling and discovering deep, causal knowledge, including the internal structure and components behavior of analyzed systems. Such a deep causal knowledge allows for different modes of Model-based Reasoning: deduction can be used to model expected system behavior, abduction can be used for analysis and diagnostic reasoning, and consistency-based reasoning can be used for structure discovery. As a tool we employ Constraint Programming. It seems that the presented approach can contribute to an interesting extension of the current Machine Learning capabilities.
Antoni Ligęza graduated from Faculty of Electrical Engineering, Automatics and Electronics (present: Faculty of Electrical Engineering, Automatics, Informatics and Electronics, EAIiE), AGH – University of Science and Technology in Cracow, Poland; received M.Sc. in electronics/automatic control in 1980. After completing Doctors Studies he received his Ph.D. degree in computer science (1983), and the habilitation (docent degree; polish Dr habilitowany) in 1994 in Computer Science/Artificial Intelligence, both from the EAIiE Faculty at AGH. In 2006 he received the professor title from the President of Poland. His main research concern Knowledge Engineering (Artificial Intelligence) including knowledge representation and inference methods, rule-based systems, automated plan generation, technical diagnostics, logics and systems science. Some most important original research results include development of backward plan generation model (1983), independent discovery of dual resolution method for automated inference (1991), the concepts of granular sets and relations (2000), granular attributive logic (2003) and diagnostic inference models in the form of logical AND/OR/NOT causal graphs (1995) and Potential Conflict Structures (1996). He was visiting professor at LAAS, Toulouse, France (1992, 1996), Universite de Nancy I, France (1994), University of Balearic Islands, Spain (1994, 1995, 2005), University of Girona, Spain (1996, 1997), and Universite de Caen, France (2004, 2005, 2007). He published (as author and co-author) more than 200 research papers, including recent monograph Logical Foundations for Rule-Based Systems”, Springer, 2006. Member of IEEE Computer Society and ACM.
Ostap Okhrin (Dresden University of Technology, Germany): Estimations of the Hierarchical Archimedean Copula
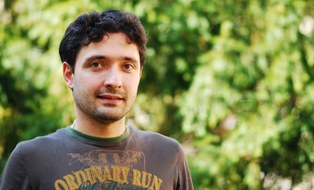
We discuss several estimators, of the hierarchical Archimedean copulas. In one, we propose the estimation of parametric hierarchical Archimedean copula while imposing an implicit penalty on its structure. Asymptotic properties of this sparse estimator are derived and issues relevant for the implementation of the estimation procedure are discussed. In the other method we propose the estimator, that uses cluster algorithm in order to obtain the structure and the parameters. Third method is based on the maximum likelihood technique. This talk is based on the several papers together with Y. Okhrin, W. Schmid, A. Ristig, A. Tetereva.
Ostap Okhrin (born 1984) studied mathematics (B.Sc. in 2004) and statistics (M.Sc. in 2005) at the Ivan Franko National University in Lviv, Ukraine. In 2008 he defended his PhD thesis at the Europa Universität Viadrina in Frankfurt (Oder) and was appointed as the Assistant Professor at the Humboldt-Universität zu Berlin. Prior his appointment at the TU Dresden he was an Associate Professor at the Humboldt-Universität zu Berlin (2014-2015). Since 2011 he made research stays at different international universities, f.e. SWUFE (Chengdu, China), Vienna University (Austria), Princeton University (USA), University of Chicago (USA), Michigan University (USA). Ostap Okhrin is in the editorial boards of four international Journals as well as author of articles in journals as Journal of the American Statistical Association, Journal of Econometrics, Econometric Theory, etc. He specialized in the multivariate distributions esp. copulas, their properties and applications in various fields from weather, over insurance to high-frequency data.